A Virtual Laboratory for E-Fulfillment Planning
Order acceptance and vehicle routing can be combined in an integrated approach. Considering delivery time windows as a scarce resource, time slot allocation on order acceptance can be controlled through revenue management and vehicle routing techniques. The aim is to maximize overall revenue from order values while supporting cost-efficient order delivery.
To benchmark the potential of iterative and integrated planning approaches, both an application-oriented catalogue of problem instances and a complex testing environment are necessary.
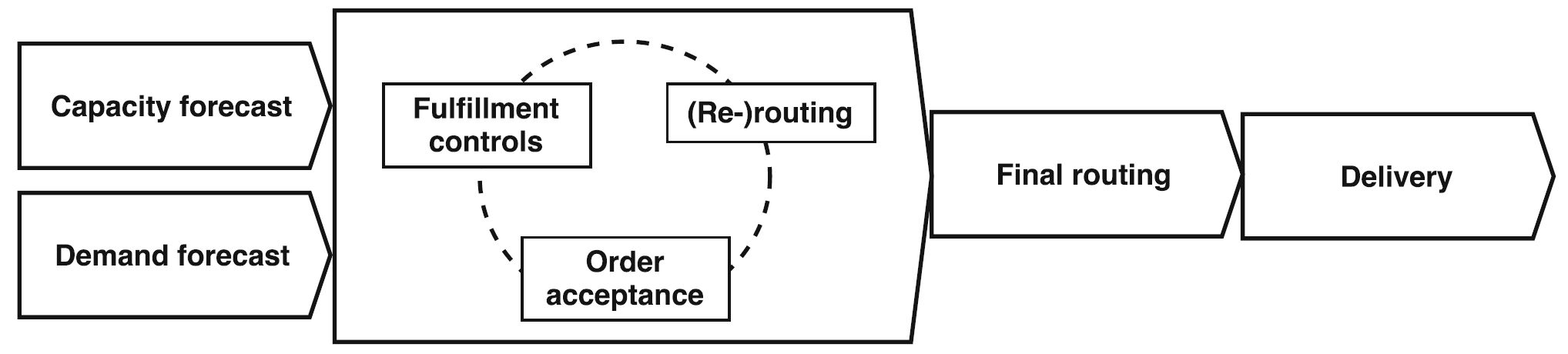
Selecting the best suited planning approach requires to examine the impact of different degrees of integration using a data-driven procedure. In an empirical case study, results can be affected by uncontrollable or unobservable factors. However, a simulation system can serve as a virtual laboratory to conduct computational studies under realistically complex settings. By implementing a library of novel and established approaches, such a laboratory allows us to evaluate and benchmark alternativ solutions. Additionally, the included simulation system enables stochastic microlevel sensitivity analyses over the impact of influence factors on all performance areas. Thereby, we can compare different scenarios entirely based on parameterized settings and methods. Appropriate data management ensures the reproducibility of results.
The figure above illustrates the high-level structure of the virtual laboratory. The idea is to embed an e-fulfillment system in a framework of complex traffic and demand behavior. As such, the e-fulfillment system is conceptually encapsulated and independent; it could readily be extracted for a stand-alone implementation in an empirical case study.
Any conceivable e-fulfillment system implements a specific process from order capture and delivery planning to recording of delivery data, with different degrees of iterative to fully integrated approaches. To ensure adaptability and extensibility, the system understands a process as a flexible orchestration of multiple, reusable methods. For instance, in the iterative variant of Cleophas and Ehmke [Cleophas, C., Ehmke, J.F.: When are deliveries profitable? Bus. Inf. Syst. Eng. 6(3), 153–163(2014)], a routing method is applied both in the initial capacity estimation and in the final step. We treat routing as an encapsulated module that can be invoked as a service from any step of the process and provides different algorithms. This service is defined by its input and output parameters. Such a service-oriented design avoids redundancies within and between alternative process implementations and supports maintainability. To allow for realistic experimental settings, real-world data has to be analyzed to calibrate the system. In particular, we will rely on historical data provided by a major e-grocery provider based in Germany. The included transactional data provides insights on customer demand such as basket value distributions and time window choice.
Since travel times in metropolitan areas vary for different daytimes, we consider the time-dependent variant of the VRPTW [Ehmke, J.F., Campbell, A.M.: Customer acceptance mechanisms for home deliveries in metropolitan areas. Eur. J. Oper. Res. 233(1), 193–207 (2014), Ehmke, J.F., Steinert, A., Mattfeld, D.C.: Advanced routing for city logistics service providers based on time-dependent travel times. J. Comput. Sci. 3(4), 193–205 (2012)]. To determine time-dependent travel times between customers, we want to include real traffic data from online map providers.
The resulting application-oriented experimental settings ensure the practical relevance of obtained insights. In addition, they can serve as starting point for alternate constraints and sensitivity analyses. For instance, we can observe effects of different degrees of heterogeneity in order basket value
